Pathfinder (2024) is a project at LAB in collaboration with partners around Europe. One of the goals is to develop AI literacy among both teachers and students. An important part of that skill is the understanding of the limitations of AI, especially when the tools can be fooled.
After the US Supreme Court decision moved the question of legality of abortions to the state level, cismen in various states started to use period tracking apps. The point is that by creating datapoints that are not factual and thus something that makes it harder for algorithms to learn from, it will make it harder for prosecutors to use the data to identify people who might have had abortions. (Docter-Loeb 2022.)
Junk data is usually defined as data that currently serves no purpose (Satori 2022) or data that is not properly managed (Adams 2022), but in the age of generative artificial intelligence a new way of thinking has risen. There are many people who do not want to participate in or contribute to the advancement of AI for various ideological or practical reasons. Thus, certain groups now see junk data as a positive, a way to protect oneself or vulnerable groups.
Wherever we create data, we can also create junk data
All our actions on the internet produce data. So much so that each second on average on the Internet produces 1.7MB of data (Delta Edge 2024). That is only our Internet activity. We also produce data by paying for things, during most of the work we do and just by walking around.
There are browser extensions that either purportedly help in some tasks, such as web development, but can be used to produce junk data or exist openly for this purpose. For example, Fake Filler is a tool for filling forms for testing purposes, but there is nothing to stop a user to use to produce junk data. Add-ons, such as AdNauseam (Howe 2024), TrackMeNot (Howe et al. 2019) and WhatCampaign (c0debabe 2020) are specifically designed to obfuscate user data by either altering information randomly or injecting data into the tracking systems.
However, junk data can also be produced in the physical world. Adversarial Fashion (2024) is both a clothing brand and an approach to fashion where the intention is to confuse facial recognition software by introducing elements that make it harder to identify a person or alternatively add false datapoints, such as random car register numbers, into the system. Sometimes these fashion brands are even working with facial recognition companies, such as Cap_able (2024a) with NtechLab (Burt 2023).
![[Alt text: marketing images of clothes and models.]](https://blogit.lab.fi/labfocus/wp-content/uploads/sites/8/2025/01/21_2025_Junk-data-the-potential-next-challenge-for-generative-AI-1024x467.jpg)
Does this work?
Whether these approaches actually do anything is dependent on the situation. For example, NtechLab stated that despite Cap_able’s attempts at dodging facial recognition with their clothing designs, they were able to positively identify each test subject (Burt 2023). The reason is that different systems use different methods for identification, so designs made for specific technology might not work against other systems.
As Docter-Loeb (2022) points out, fake data in a period tracking system can only help under specific circumstances. If the record for a specific suspect is subpoenaed, data provided by other users does not matter. Also, if prosecutors attempt to data mine for batches of data, this approach is helpful only if there is no pattern to find or the fake accounts can fake that pattern. Otherwise, the system will simply filter out these attempts as outliers unless there are enough of them, but that would require millions of fake users.
The data being gathered is rarely used to understand a specific user. It is more about building profiles for a specific reference group and only a small portion of that group needs to provide valid data for that to be possible, so personal decisions regarding how you share data are often meaningless.
Author
Aki Vainio works as a senior lecturer of IT at LAB and takes part in various RDI projects in expert roles. He did not intend this as a guide.
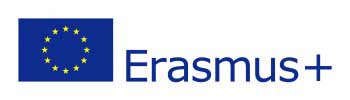
References
Adams, D. 2022. Moving From “Junk” Data to Data Integrity. Database Trends and Applications. Cited 7 Jan 2025. Available at https://www.dbta.com/Editorial/News-Flashes/Moving-From-Junk-Data-to-Data-Integrity-151759.aspx
Adversarial Fashion. 2024. Surveillance Self-defense & Other Actions You Can Take.
Burt, C. 2023. Designers take on facial recognition with adversarial fashion. Biometric Update.com. Cite 7 Jan 2025. Available at https://www.biometricupdate.com/202302/designers-take-on-facial-recognition-with-adversarial-fashion
c0debabe. 2020. WhatCampaign. Firefox Add-Ons. Cited 19 Dec 2024. Available at https://addons.mozilla.org/en-US/firefox/addon/whatcampaign
Cap_able. 2024a. Technology | Adversarial Fashion. capable.design. Cited 7 Jan 2025. Available at https://www.capable.design/blogs/notizie/technology-adversarial-fashion
Cap_able. 2024b. Products. capable.design. Cited 19 Dec 2024. Available at https://www.capable.design/collections/all
Docter-Loeb, H. 2022. Can Men Downloading Period Apps Help Protect People Seeking Abortions?. Slate. Cited 7 Jan 2025. Available at https://slate.com/technology/2022/07/men-period-tracking-apps-abortion.html
Edge Delta. 2024. Breaking Down The Numbers: How Much Data Does The World Create Daily in 2024?. Edge Delta. Cited 7 Jan 2025. Available at https://edgedelta.com/company/blog/how-much-data-is-created-per-day
Fake Filler. 2024. Chrome Web Store. Cited 19 Dec 2024. Available at https://chromewebstore.google.com/detail/Fake%20Filler/bnjjngeaknajbdcgpfkgnonkmififhfo
Howe, D. 2024. AdNauseam. Firefox Add-Ons. Cited 19 Dec 2024. Available at https://addons.mozilla.org/en-US/firefox/addon/adnauseam/
Howe, D., Nissenbaum, H. & Janoss. 2019. TrackMeNot. Firefox Add-Ons. Cited 19 Dec 2024. Available at https://addons.mozilla.org/en-US/firefox/addon/trackmenot/
Pathfinder. 2024. Welcome to the Erasmus+ Pathfinder Project. Netlify. Cited 7 Jan 2025. Available at https://erasmus-pathfinder.netlify.app/
Satori. 2022. Junk Data. Satori Cyber Ltd. Cited 7 Jan 2025. Available at https://satoricyber.com/glossary/junk-data/