Peeling knives are crucial components in the plywood manufacturing process. They determine the quality and efficiency of veneer production that is used to make plywood. Peeling knives are involved in processes of removing the bark from a log and peeling off the useful volume of a wooden block into a thin veneer (Raute 2023a).
A dull or damaged knife can result in reduced yield, increased energy consumption, and lower product quality (Raute 2023b). When a knife is not sharp, it can cause breaks in the veneer ribbon or create waviness in the veneer ribbon, resulting in lower quality or even unusable veneer slices. Furthermore, a dull knife requires more force to peel the log, which leads to higher energy consumption and increased wear and tear on the surrounding equipment of the machine.
Currently, the sharpness of peeling knives is often checked visually by operators based on quality of the produced veneer ribbon. This process is reactive and subjective, as different operators may have different standards for what constitutes a sharp knife. Moreover, manual inspections to detect changes in knife sharpness led to cost losses by decreased product quality. (Lutai 2023.)
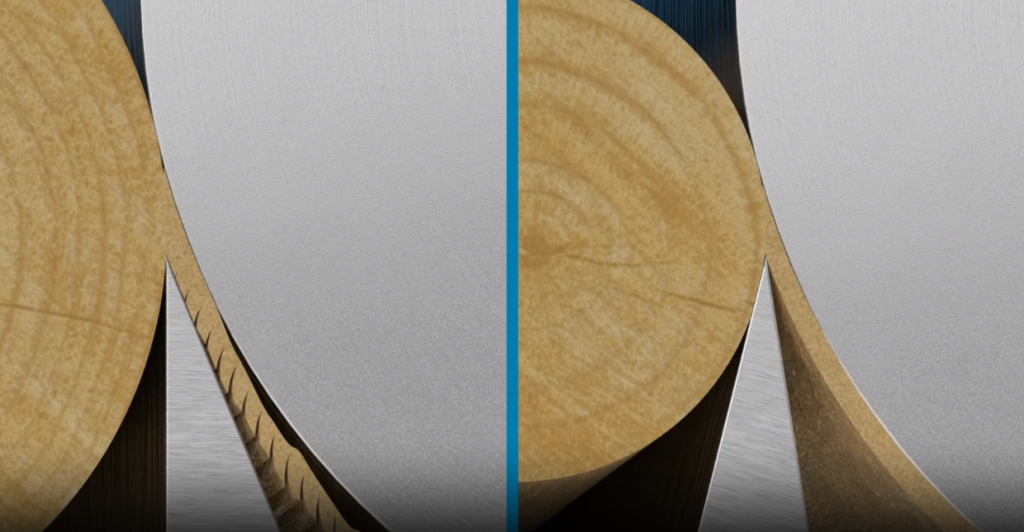
Unleashed Operational Efficiency Driven by Data
Data-driven analysis can be applied to the data from the peeling process to identify patterns and trends to predict when the peeling knife requires maintenance or replacement. Similar studies were conducted by Roman Lutai in his thesis work for LAB. This work studied correlations between production data collected and the lifespan of a knife. This task was employing machine learning tools. (Lutai 2023.) Findings could provide the base for the developing of a proactive automated notification system.
Data-driven patterns can be translated into estimations of expected kilometers that the peeling can peel. Providing such information to operators would relieve them from responsibility to monitor the knife quality and to make decisions of its maintenance. It allows operators to concentrate more on the peeling process. Moreover, knife status insights would enable operators to optimize operational parameters with increased accuracy.
Alongside with operators’ view, this solution would benefit also the maintenance team. Predictions of knife status would enable proactive maintenance, allowing clear schedule planning as well as providing time to prepare tools and spare parts without hurry. It would not only decrease the duration of maintenance downtime, but also prevent surrounding parts of the peeling line from excessive wear due to a dull knife. Moreover, unnatural drop in predicted knife status could provide meta-knowledge about issues in the installation overall.
Combining all together, such solution would lead to multiple benefits by decreasing several negative time properties. The duration of downtimes would decrease due to proactive maintenance actions. The time of lower quality production due to the wear off of the knife would decrease based on valuable insights available to operators, enabling the yield of a higher-quality product.
These benefits are leading not only to increased productions capacities and qualities hence profitability, but also are decreasing the amount of waste produced. Therefore, these improvements would not only lead to better sustainability in production but also resonate with environmentally conscious ideas and align with responsible forestry practices.
Authors
Roman Lutai is a student in the “From IoT to AI” Master’s programme in engineering at LAB University of Applied Sciences.
Minna Asplund is a senior lecturer in Information and Communications Technology. She is also the coordinator of the “From IoT to AI” Master’s programme in engineering at LAB University of Applied Sciences.
References
Lutai, R. 2023. Data-driven knife sharpness meter for peeling lines. Master’s thesis. LAB University of Applied Sciences, Faculty of Technology. Lahti. Cited 15 Nov 2023. Available at: https://urn.fi/URN:NBN:fi:amk-2023111329227
Raute. 2023a. Optimal Peeling Maximizes Veneer Quality and Production Capacity. Cited 15 Nov 2023. Available at: https://www.raute.com/lines-and-machines/lines/veneer-peeling/
Raute. 2023b. Optimal peeling geometry (OPG). Cited 15 Nov 2023. Available at: https://materials.raute.com/file/dl/i/zGvVaA/-fMEyAdXqnxdkuBAMvpJfg/Raute-Optimal-Peeling-Geometry-animation-en.mp4?t=1625472718