Artificial Intelligence (AI) has emerged as a transformative force in various fields, including leadership and management. AI represents a distinct form of intelligence, particularly in its ability to share knowledge across multiple systems instantly—something humans cannot do. By leveraging AI, leaders can enhance decision-making, improve organizational efficiency, and foster a more innovative work environment wherein the continuous development and implementation of new ideas flourish. This, in turn, can lead to further innovations (Schumpeter 1934) and competitive advantages (Porter 1980). Innovations are essential to sustaining long-term organizational success.
AI and data are becoming key drivers for innovation and significantly impact the innovation process and innovation management aspects (Tekic & Füller 2023). AI has changed and continues to influence decision-making and innovation within organizations (Pietronudo et al. 2022). Advanced AI systems, such as predictive AI, generative AI, and agentic AI, enable leaders to anticipate trends, optimize strategies, and automate complex decision-making processes. Predictive AI involves applying statistical models, machine learning, and historical data analysis to forecast future events or outcomes. Generative AI refers to algorithms designed to produce original content, like text, code, images, video, or audio, by learning patterns from existing data. (Božić 2023.) Agentic AI refers to systems capable of autonomously pursuing complex goals with minimal human supervision, often through multi-step decision-making and adaptive behavior. (Shavit et al. 2023.) Business innovation in the coming era will be driven by the orchestration of these and other AI systems working in concert.
Moreover, AI-driven collaboration tools facilitate cross-border innovation, allowing geographically dispersed teams to work together effectively with limited resources (Enkel et al. 2020; Kanakaris et al. 2019). The increased need for both data and new technology, combined with a shrinking workforce due to demographic shifts, necessitates the adoption of open, AI-augmented collaborative innovation processes. AI-powered knowledge graphs, intelligent automation, and NLP-based virtual assistants are becoming integral to knowledge management and organizational learning. AI-powered knowledge graphs are structured representations of entities and their relationships, enhanced by artificial intelligence techniques that support their automated construction, enrichment, and reasoning capabilities. (Hogan et al. 2021.) AI-powered intelligent automation refers to the combination of robotic process automation and artificial intelligence, enabling more advanced, adaptable, and efficient process automation across organizations. (Ng et al. 2021.) NLP-based virtual assistants are AI-driven systems that use natural language processing to interpret user input and generate contextually relevant responses, enabling interactive, human-like communication. (Park 2023; Hoy 2018.) Together, these AI-driven technologies are accelerating digital transformation by enhancing organizational agility, streamlining collaboration, and unlocking a sustained competitive advantage in the race for innovation.
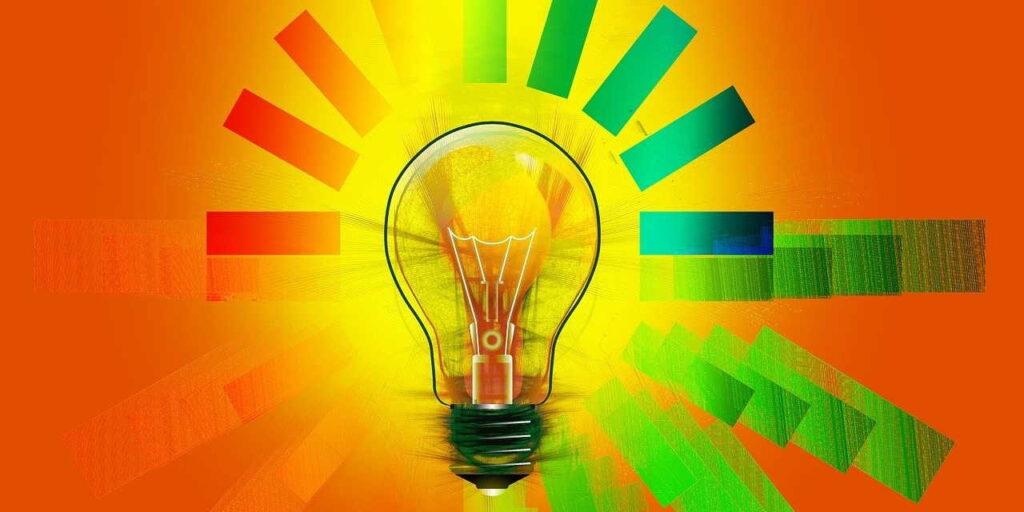
AI-Enhanced Innovation Management & Challenges
As AI becomes embedded in innovation teams, it plays a crucial role in accelerating R&D, enhancing product design, and streamlining operations (Siakas et al. 2016; Makkonen et al. 2023; Brusila-Meltovaara et al. 2024). However, despite these benefits, organizations often fail to fully harness AI-driven innovation due to technical and organizational barriers. These challenges are presented in Table 1.
Challenge | Effect on AI-Driven Innovation |
Data silos and integration issues | Limits AI’s ability to access and analyze comprehensive, high-quality data, reducing the accuracy and usefulness of insights. |
Skill gaps in AI and data science | Prevents effective development, deployment, and maintenance of AI systems, which slows innovation and adoption. |
Ethical and regulatory constraints | Introduces compliance hurdles; demands explainability, fairness, and transparency, which can delay implementation or limit certain use cases. |
Ineffective collaboration with external AI experts | Leads to missed opportunities for knowledge transfer, innovation, and capability building, resulting in underutilized AI potential. |
To overcome these barriers, organizations must invest in AI literacy, foster interdisciplinary collaboration, and build AI governance frameworks to ensure responsible and effective AI use in innovation management (Hoy 2018). Inclusion of the aforementioned in the curricula of university students provides the basis for equipping future professionals with the necessary skills and knowledge to navigate and leverage AI in their respective fields. By fostering a comprehensive understanding of AI and interdisciplinary collaboration, universities can prepare students to drive responsible and innovative AI applications in their careers, ultimately contributing to the advancement and ethical management of AI technologies in various industries.
In parallel, industry must implement structured onboarding platforms that align employee engagement with organizational AI governance strategies. These platforms should operationalize principles and policies that foster digital trust, ensuring that AI adoption is not only technically proficient but also ethically grounded. By embedding governance into practice from the outset, organizations can create a culture of accountability and stewardship, reinforcing responsible innovation and public confidence in AI-enabled systems.
Authors
Kristiina Brusila-Meltovaara works as a principal lecturer in Faculty of Technology at LAB University of Applied Sciences.
Christine Thomson of Zitiso Inc. is an AI consultant and advisor to Fortune 500 companies, supporting their adoption and implementation of AI solutions.
References
Božić, V. 2023. AI and Predictive Analytics. Cited 27 Mar 2025. Available at https://www.researchgate.net/publication/370074080_AI_and_Predictive_Analytics
Brusila-Meltovaara, K., Makkonen, P., Siakas, K. & Lampropoulos, G. 2024. Open Innovation Capabilities and Competences. In Buisine, S. (ed.). Proceedings of the 19th European Conference on Innovation and Entrepreneurship ECIE 2024. Paris-Nanterre, France. 26–27 Sep 2024. Academic Conferences International. 110–117. Cited 6 Mar 2025. Available at https://doi.org/10.34190/ecie.19.1.2487
Enkel, E., Bogers, M. & Chesbrough, H. 2020. Exploring open innovation in the digital age: A maturity model and future research directions. R & D management. Vol. 50 (1), 161–168. Cited 6 Mar 2025. Available at https://doi.org/10.1111/radm.12397
Füller, J., Hutter, K., Wahl, J., Bilgram, V. & Tekic, Z. 2022. How AI revolutionizes innovation management–Perceptions and implementation preferences of AI-based innovators. Technological Forecasting and Social Change. Vol. 178, 121598. Cited 6 Mar 2025. Available at https://www.sciencedirect.com/science/article/pii/S0040162522001305
geralt. 2015. Hehkulamppu, paistaa, idea, ajatus. Pixabay. Cited 27 Mar 2025. Available at https://pixabay.com/fi/illustrations/hehkulamppu-paistaa-idea-ajatus-978882/
Hogan, A., Blomqvist, E., Cochez, M., d’Amato, C., Melo, G. D., Gutierrez, C.& Zimmermann, A. 2021. Knowledge graphs. ACM Computing Surveys (Csur). Vol. 54 (4), 1–37. Cited 6 Mar 2025. Available at https://dl.acm.org/doi/10.1145/3447772
Hoy, M. B. 2018. Alexa, Siri, Cortana, and More: An Introduction to Voice Assistants. Med Ref Serv Q. Vol. 37 (1), 81–88. Cited 6 Mar 2025. Available at https://doi: 10.1080/02763869.2018.1404391.
Kanakaris, V., Lampropoulos, G., & Siakas, K. 2019. A Survey and a Case-Study Regarding Social Media Security and Privacy on Greek Future IT Professionals. International Journal of Human Capital and Information Technology Professionals (IJHCITP). Vol. 10 (1), 22–37. Cited 6 Mar 2025. Available at https://doi.org/10.4018/IJHCITP.2019010102
Makkonen, P., Siakas, K., Lampropoulos, G. & Brusila-Meltovaara, K. 2023. Open Innovation Maturity in Companies. In Moreira, F. & Jayantilal, S. (eds.). Proceedings of the European Conference on Innovation and Entrepreneurship, Part 1. Porto, Portugal. 21–22 September. Universidade Portucalense. Vol. 18 (1), 545–551. Cited 6 Mar 2025. Available at https://doi.org/10.34190/ecie.18.1.1622
Ng, K. K. H., Chen, C., Lee, C., Jiao, R. & Yang, Z. 2021. A systematic literature review on intelligent automation: Aligning concepts from theory, practice, and future perspectives. Advanced Engineering Informatics. Vol. 47, 101246. Available at https://doi.org/10.1016/j.aei.2021.101246
Pietronudo, M. C., Croidieu, G. & Schiavone, F. 2022. A solution looking for problems? A systematic literature review of the rationalizing influence of artificial intelligence on decision-making in innovation management, Technological Forecasting and Social Change. Vol. 182,121828. Cited 5 Mar 2025. Available at https://www.sciencedirect.com/science/article/abs/pii/S0040162522003523
Park, S. H. 2023. Use of Generative Artificial Intelligence, Including Large Language Models Such as ChatGPT, in Scientific Publications: Policies of KJR and Prominent Authorities. Korean Journal of Radiology. Vol. 24 (8), 715–718. Cited 6 Mar 2025. Available at https://doi.org/10.3348/kjr.2023.0643
Porter, M. E. 1980. Industry structure and competitive strategy: Keys to profitability. Financial analysts journal. Vol. 36 (4), 30–41. Cited 6 Mar 2025. Available at https://www.jstor.org/stable/4478361
Siakas, K., Georgiadou, E. & Siakas, D. 2016. The influence of national and organisational culture on knowledge sharing in distributed teams. International Journal of E-Entrepreneurship and Innovation (IJEEI). Vol. 6 (1), 19–37. Cited 6 Mar 2025. Available at: https://doi.org/10.4018/978-1-7998-0417-8
Schumpeter, J. A. 1934. The Theory of Economic Development. Cambridge: Harvard University Press.
Shavit, Y., Agarwal, S., Brundage, M., Adler, S., O’Keefe, C., Campbell, R., Lee, T., Mishkin, P., Eloundou, T., Hickey, A., Slama, K., Ahmad, L., McMillan, P., Beutel, A., Passos, A. & Robinson, D. G. 2023. Practices for governing agentic AI systems. OpenAI Research Paper. Cited 6 Mar 2025. Available at https://cdn.openai.com/papers/practices-for-governing-agentic-ai-systems.pdf
Tekic, Z. & Füller, J. 2023. Managing innovation in the era of AI. Technology in Society. Vol. 73, 102254. Cited 27 Mar 2025. Available at https://cdn.openai.com/papers/practices-for-governing-agentic-ai-systems.pdf